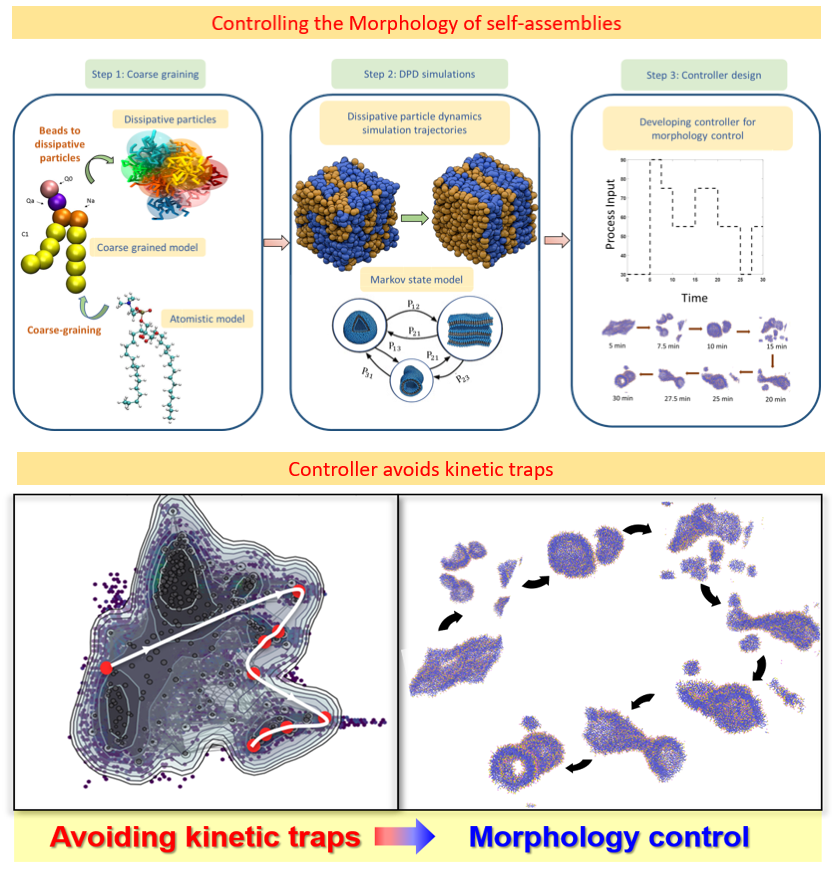
The process of self-assembly, wherein molecules come together to form intricate structures through the forces of interaction, finds wide-ranging applications across various fields. However, effectively managing the dynamics of self-assembling molecules presents challenges due to factors such as kinetic trapping, as well as the stochastic and nonlinear characteristics of the governing equations. Previous research has utilized Markov decision processes to control self-assemblies, but this approach may not be suitable for more complex systems and could lead to disassembly or irreversible changes. To overcome these limitations, a new comprehensive framework is proposed for the systematic development of control strategies for self-assembling systems. This framework utilizes a dissipative particle dynamics model to accurately capture self-assembly and generate a detailed representation of relevant nanostructures. The probabilities of transitioning between states are then calculated, facilitating the formulation of a stochastic optimal control problem. Dynamic programming is employed to solve this problem. The framework is applied to a case study involving dynamic binary complexes with multiple undesired metastable states, serving as an experimental demonstration of the controller’s effectiveness in driving the system toward desired morphologies. Ultimately, the results of closed-loop simulations underscore the capability of the proposed framework to guide thermosensitive DBC systems toward target morphologies while avoiding kinetic traps
Literature:
S. Pahari, S. Liu, Y. Lin, C. Lee, M. Akbulut, and J.S.-I. Kwon, “Stochastic optimal control of mesostructure of supramolecular assemblies using dissipative particle dynamics and dynamic programming with experimental validation”, Chem. Eng. J., 2023, Revised & Resubmitted.